Note
Go to the end to download the full example code.
Quantile regression with oblique regression forest#
An example to generate quantile predictions using an oblique random forest instance on a synthetic, right-skewed dataset.
This example was heavily inspired by quantile-forest package. See their package here.
from collections import defaultdict
import matplotlib.pyplot as plt
import numpy as np
import scipy as sp
from sklearn.model_selection import train_test_split
from sklearn.utils.validation import check_random_state
from treeple.ensemble import ObliqueRandomForestRegressor
rng = check_random_state(0)
Generate the data#
We use a synthetic dataset with 2 features and 5000 samples. The target is generated from a skewed normal distribution. (The mean of the distribution is to the right of the median.)
n_samples = 5000
a, loc, scale = 5, -1, 1
skewnorm_rv = sp.stats.skewnorm(a, loc, scale)
skewnorm_rv.random_state = rng
y = skewnorm_rv.rvs(n_samples)
X = rng.randn(n_samples, 2) * y.reshape(-1, 1)
X_train, X_test, y_train, y_test = train_test_split(X, y, test_size=0.5, random_state=0)
regr_orf = ObliqueRandomForestRegressor(n_estimators=10, random_state=0)
regr_orf.fit(X_train, y_train)
y_pred_orf = regr_orf.predict(X_test)
Generate Quantile Predictions#
The idea is for each prediction, the training samples that fell into the same leaf nodes are collected then used to generate the quantile statistics for the desired prediction.
# Get the leaf-nodes the training samples fall into
leaf_ids = regr_orf.apply(X_train)
# create a list of dictionary that maps node to samples that fell into it
# for each tree
node_to_indices = []
for tree in range(leaf_ids.shape[1]):
d = defaultdict(list)
for id, leaf in enumerate(leaf_ids[:, tree]):
d[leaf].append(id)
node_to_indices.append(d)
# drop the X_test to the trained tree and
# get the indices of leaf nodes that fall into it
leaf_ids_test = regr_orf.apply(X_test)
# for each samples, collect the indices of the samples that fell into
# the same leaf node for each tree
y_pred_quantile = []
for sample in range(leaf_ids_test.shape[0]):
li = [
node_to_indices[tree][leaf_ids_test[sample][tree]] for tree in range(leaf_ids_test.shape[1])
]
# merge the list of indices into one
idx = [item for sublist in li for item in sublist]
# get the y_train for each corresponding id
y_pred_quantile.append(y_train[idx])
# get the quatile preditions for each predicted sample
y_pred_quantile = [np.quantile(y_pred_quantile[i], 0.5) for i in range(len(y_pred_quantile))]
Plot the results#
The plot shows the distribution of the actual target values and the predicted median (i.e. 0.5 quantile), and the mean prediction by the regular random forest regressor. In this skewed dataset, the median prediction using the quantile method works better at predicting the off-centered target distribution than the regular mean prediction.
colors = ["#c0c0c0", "#a6e5ff", "#e7a4f5"]
names = ["Actual", "QRF (Median)", "ORF (Mean)"]
plt.hist([y_test, y_pred_quantile, y_pred_orf], bins=50, color=colors, label=names)
plt.xlabel("Actual and Predicted Target Values")
plt.ylabel("Counts")
plt.legend()
plt.show()
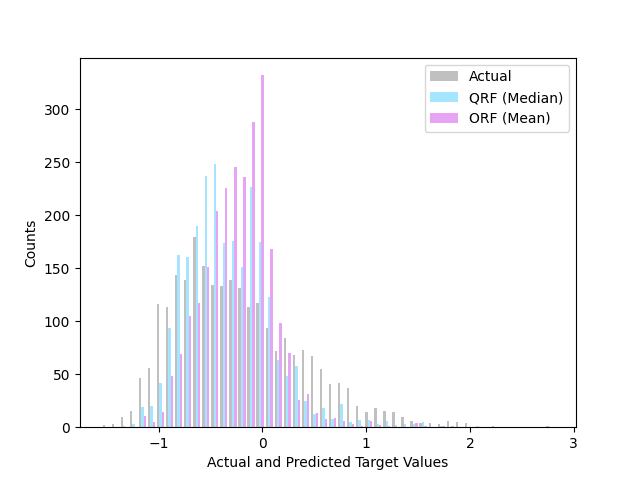
Total running time of the script: (0 minutes 1.346 seconds)
Estimated memory usage: 224 MB