Note
Go to the end to download the full example code.
ExtendedIsolationForest example#
An example using ExtendedIsolationForest
for anomaly
detection, which compares to the IsolationForest
based
on the algorithm in [1].
In the present example we demo two ways to visualize the decision boundary of an Extended Isolation Forest trained on a toy dataset.
Data generation#
We generate two clusters (each one containing n_samples
) by randomly
sampling the standard normal distribution as returned by
numpy.random.randn()
. One of them is spherical and the other one is
slightly deformed.
For consistency with the IsolationForest
notation,
the inliers (i.e. the gaussian clusters) are assigned a ground truth label 1
whereas the outliers (created with numpy.random.uniform()
) are assigned
the label -1
.
from copy import copy
import matplotlib.pyplot as plt
import numpy as np
from sklearn.ensemble import IsolationForest
from sklearn.inspection import DecisionBoundaryDisplay
from sklearn.model_selection import train_test_split
from treeple import ExtendedIsolationForest
n_samples, n_outliers = 120, 40
rng = np.random.RandomState(0)
covariance = np.array([[0.5, -0.1], [0.7, 0.4]])
cluster_1 = 0.4 * rng.randn(n_samples, 2) @ covariance + np.array([2, 2]) # general
cluster_2 = 0.3 * rng.randn(n_samples, 2) + np.array([-2, -2]) # spherical
outliers = rng.uniform(low=-4, high=4, size=(n_outliers, 2))
X = np.concatenate([cluster_1, cluster_2, outliers])
y = np.concatenate([np.ones((2 * n_samples), dtype=int), -np.ones((n_outliers), dtype=int)])
X_train, X_test, y_train, y_test = train_test_split(X, y, stratify=y, random_state=42)
We can visualize the resulting clusters:
scatter = plt.scatter(X[:, 0], X[:, 1], c=y, s=20, edgecolor="k")
handles, labels = scatter.legend_elements()
plt.axis("square")
plt.legend(handles=handles, labels=["outliers", "inliers"], title="true class")
plt.title("Gaussian inliers with \nuniformly distributed outliers")
plt.show()
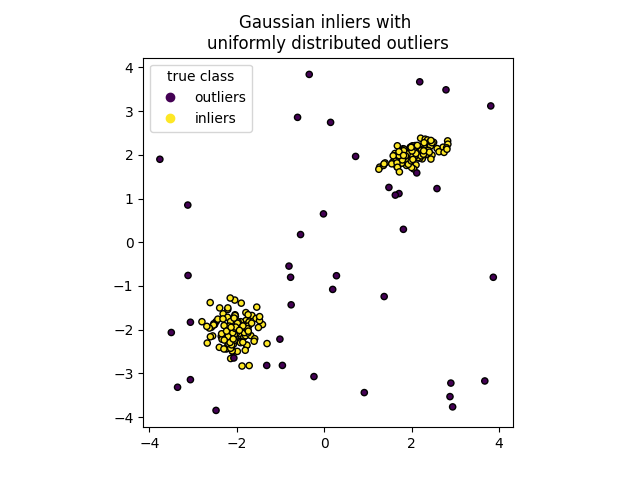
Training of the model#
extended_clf = ExtendedIsolationForest(max_samples=100, random_state=0, feature_combinations=2)
extended_clf.fit(X_train)
clf = IsolationForest(max_samples=100, random_state=0)
clf.fit(X_train)
Plot discrete decision boundary#
We use the class DecisionBoundaryDisplay
to
visualize a discrete decision boundary. The background color represents
whether a sample in that given area is predicted to be an outlier
or not. The scatter plot displays the true labels.
for name, model in zip(["IsoForest", "ExtendedIsoForest"], [clf, extended_clf]):
disp = DecisionBoundaryDisplay.from_estimator(
model,
X,
response_method="predict",
alpha=0.5,
)
disp.ax_.scatter(X[:, 0], X[:, 1], c=y, s=20, edgecolor="k")
disp.ax_.set_title(f"Binary decision boundary \nof {name}")
plt.axis("square")
plt.legend(handles=handles, labels=["outliers", "inliers"], title="true class")
plt.show()
Plot path length decision boundary#
By setting the response_method="decision_function"
, the background of the
DecisionBoundaryDisplay
represents the measure of
normality of an observation. Such score is given by the path length averaged
over a forest of random trees, which itself is given by the depth of the leaf
(or equivalently the number of splits) required to isolate a given sample.
When a forest of random trees collectively produce short path lengths for
isolating some particular samples, they are highly likely to be anomalies and
the measure of normality is close to 0
. Similarly, large paths correspond to
values close to 1
and are more likely to be inliers.
for name, model in zip(["IsoForest", "ExtendedIsoForest"], [clf, extended_clf]):
disp = DecisionBoundaryDisplay.from_estimator(
model,
X,
response_method="decision_function",
alpha=0.5,
)
disp.ax_.scatter(X[:, 0], X[:, 1], c=y, s=20, edgecolor="k")
disp.ax_.set_title(f"Path length decision boundary \nof {name}")
plt.axis("square")
plt.legend(handles=handles, labels=["outliers", "inliers"], title="true class")
plt.colorbar(disp.ax_.collections[1])
plt.show()
Generate Data Produce 2-D dataset with a sinusoidal shape and Gaussian noise added on top.
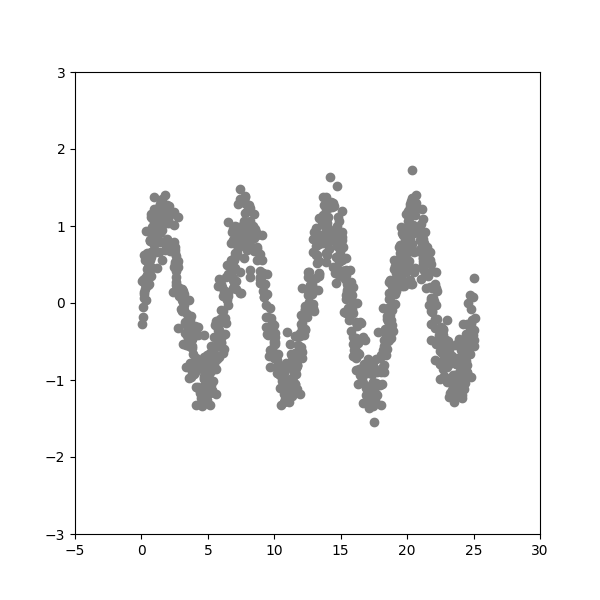
extended_clf = ExtendedIsolationForest(
max_samples=100, random_state=0, n_estimators=200, feature_combinations=2
)
extended_clf.fit(X)
clf = IsolationForest(max_samples=100, random_state=0, n_estimators=200)
clf.fit(X)
Plot discrete decision boundary
for name, model in zip(["IsoForest", "ExtendedIsoForest"], [clf, extended_clf]):
disp = DecisionBoundaryDisplay.from_estimator(
model,
X,
response_method="decision_function",
alpha=0.5,
)
disp.ax_.scatter(X[:, 0], X[:, 1], c=y, s=15, edgecolor="k")
disp.ax_.set_title(f"Path length decision boundary \nof {name}")
plt.colorbar(disp.ax_.collections[1])
plt.show()
Visualize the prediction of each tree within the forest#
We can visualize the prediction of each tree within the forest by using a circle plot and showing the depth at which each tree isolates a given sample. Here, we will evaluate two samples in the sinusoidal plot: one inlier and one outlier. The inlier is located at the center of the sinusoidal and the outlier is located at the bottom right corner of the plot.
inlier_sample = np.array([10.0, 0.0])
outlier_sample = np.array([-5.0, -3.0])
for name, model in zip(["IsoForest", "ExtendedIsoForest"], [clf, extended_clf]):
theta = np.linspace(0, 2 * np.pi, len(model.estimators_))
max_tree_depth = max([model.estimators_[i].get_depth() for i in range(len(model.estimators_))])
fig = plt.figure()
ax = plt.subplot(111, projection="polar")
radii_in = []
radii_out = []
# get the depth of each samples
for radii, sample, color, lw, alpha in zip(
[radii_in, radii_out], [inlier_sample, outlier_sample], ["b", "r"], [1, 1.3], [1, 0.9]
):
for i in range(len(model.estimators_)):
# get the max depth of this tree
max_depth_tree = model.estimators_[i].get_depth()
leaf_index = model.estimators_[i].apply(sample.reshape(1, -1))
# get the depth of each tree's leaf node for this sample
depth = model._decision_path_lengths[i][leaf_index].squeeze()
radii.append(depth)
radii = np.array(radii)
radii = np.sort(radii) / max_tree_depth
for j in range(len(radii)):
ax.plot([theta[j], theta[j]], [0, radii[j]], color=color, alpha=alpha, lw=lw)
if color == "b":
radii_in = copy(radii)
else:
radii_out = copy(radii)
ax.set(
title=f"{name}\nNominal: Mean={np.mean(radii_in).round(3)}, "
f"Var={np.var(radii_in).round(3)}\n"
f"Anomaly: Mean={np.mean(radii_out).round(3)}, Var={np.var(radii_out).round(3)}",
xlabel="Anomaly",
)
ax.set_xticklabels([])
ax.axes.get_xaxis().set_visible(False)
ax.axes.get_yaxis().set_visible(False)
fig.tight_layout()
plt.show()
References#
Total running time of the script: (0 minutes 8.031 seconds)
Estimated memory usage: 237 MB